The Challenge
Throughout 2021, the client changed business direction and YLD faced the challenge of adapting its support to align with the shifts in the client's strategy and focus.
The Approach
From providing the most accurate prediction for money-saving opportunities to automating customer service inquiries without compromising quality, our team has reached numerous milestones along the way.
After changes in business direction, we remained committed to supporting the client and its customers, working tirelessly to ensure a smooth transition during the sale. We have been working with them across the following key areas:
- Wholesale teams
- Customer-focused teams
Breaking new ground
We worked closely with the energy supplier using agile methodology and held weekly meetings to discuss goals and priorities with the client's key stakeholders. Both teams also had daily check-ins to ensure everyone was aligned to keep the project moving forward.
This project tackled major areas impacting the energy supplier itself and prospective customers of the platform. Below are highlights of the work that’s been done during the partnership with the client:
1. Reducing Risk and Driving Innovation with YLD's Machine Learning Model
Typically, energy companies use rough estimates for consumer usage and provide these estimates back to the consumer, but these estimates often need to be more accurate and can lead to inefficiencies and increased costs.
To minimise inaccuracies, we incorporated machine learning into the forecasting process. We developed a proof of concept machine learning model to test the waters that challenged the industry standard algorithm “Mean Absolute Percentage Error” (MAPE). The goal of the model was to help the client spend their daily wholesale budget more efficiently. The graph shows how well YLD's model predicts energy usage compared to the actual amount used.
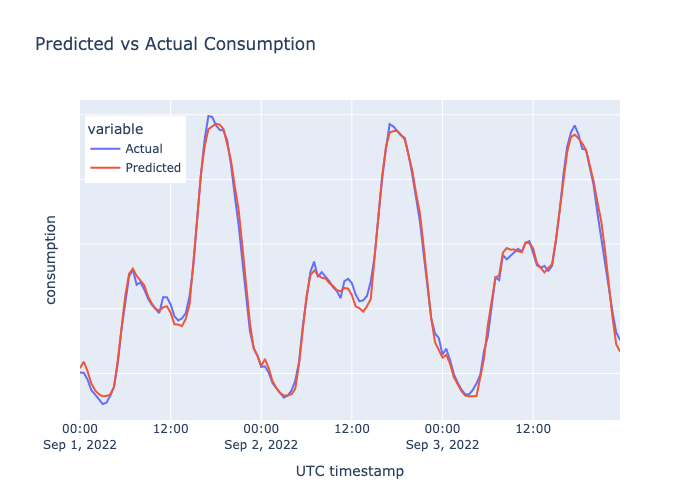
After deploying the proof of concept, the machine learning model MAPE outperformed the industry standard average, reducing the error rate by 0.86%.
2. Disrupting Customer Service with Machine Learning and Automation
One of the main missions of this project was to reduce customer service operations costs while maintaining a high level of user satisfaction. To achieve this, we worked closely with the client teams to further leverage machine learning by identifying the reasons behind customer inquiries and building a bot that can respond with the same quality and efficiency as a human.
One of the milestones the team achieved was reducing the inflow of customer inquiries by 30% by training the bot on common tickets, i.e. requests from customers to update their meter readings.
3. Predicting Monetary Savings with Machine Learning
Homeowners often receive recommendations and estimated monetary savings from Energy Performance Certificates (EPCs) regarding energy-efficient upgrades. However, transferring this information to the customer through the client’s app was difficult due to missing API values.
Our teams and the client tackled this challenge by analysing trends and developing an efficient and accurate system for monetary savings estimation and individual recommendations.
As a result, the platform now has an advanced feature that crafts and provides personalised recommendations to end-users based on their energy usage and upgrade opportunities.
We saw this as an opportunity for end-users to have a personal EPC surveyor at the palm of their hands to provide valuable insights and savings opportunities.
4. Combatting Energy Theft with Data Analytics and ML
Energy theft is a massive issue in the energy sector, causing significant inconvenience for customers paying the bill. On top of this, house inspections can be expensive for customers and disruptive to day-to-day activities. To combat this problem, our experts developed a sophisticated screening model that leverages data analytics and machine learning to detect and identify the source and kinds of specific indicators of energy theft.


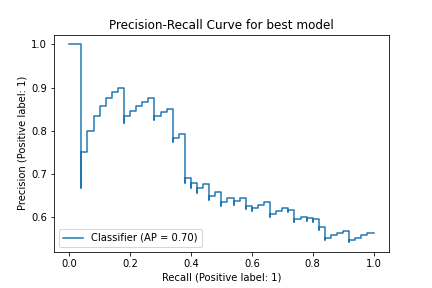
The graph presents an average precision score of 0.851, indicating that it is usually correct every time a model predicts a theft. Equally, a 29% improvement over random selection can be achieved using machine learning, and the Cross Validation score of 0.79 indicates that a robust model has been created.
The model seeks specific scenarios, like a sudden increase in energy consumption and/ or abnormal top-ups on customer accounts, among others. It acts as an early warning system that alerts the energy provider of suspicious activities, helping prevent energy theft from occurring in the first place.
People have heard that prevention is better than cure, and in light of preventing energy theft, our teams took a step further and created a pre-screening model to spot theft even before it occurs. The model uses SQL for data pre-processing and Google Collab to model and help identify the most prominent indicators of energy theft before it takes place.
It’s vital to note that expecting energy theft before it occurs is a far more challenging problem as actual energy thefts are rare - only 0.0117% of all accounts have associated energy theft. Impressively, while in its early stages, the model has identified a remarkable 63% of all actual energy thefts by generating synthetic data (SMOTE).
The Deliverables
We helped boost the client’s data capabilities by infusing data science and analytical expertise into teams, tackling practical and major activities impacting the end-user.
Our successful collaboration resulted in a 0.86% error rate decreased and a 30% payment forecast accuracy improved.
Closing the Engagement
Through partnering with YLD, our client successfully harnessed the power of data science and machine learning to tackle multifaceted challenges. With a shared vision of streamlining energy accessibility and affordability, we worked together to provide consumers with enhanced insights into their energy usage and to automate operational processes for optimal efficiency.